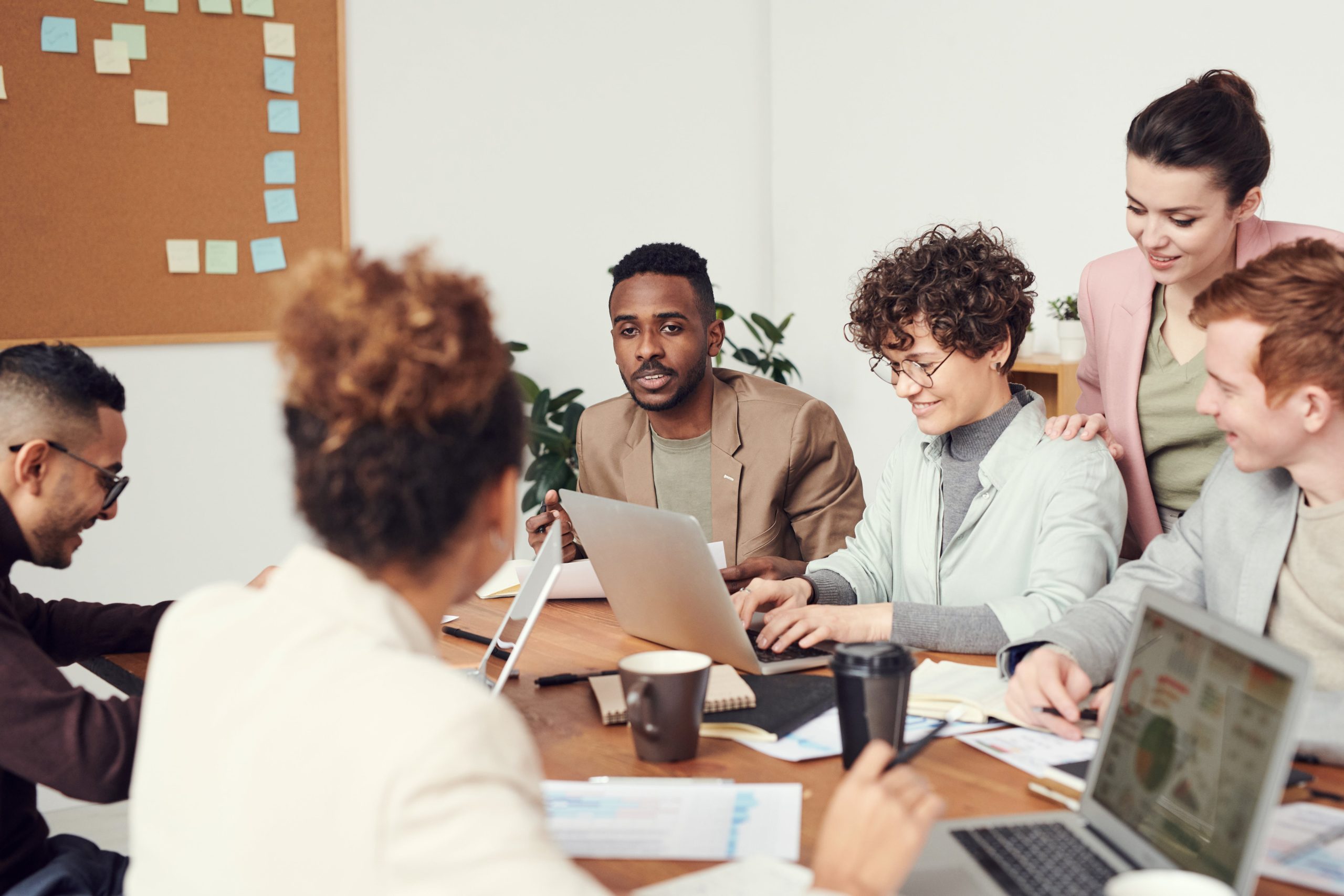
Bias and Discrimination in Artificial Intelligence
The Civil Rights Act of 1964 was signed into law by President Lyndon B. Johnson to prohibit discrimination. While people and organizations have made great strides in the almost sixty years since that law was passed, the rise of artificial intelligence (AI) technology has introduced new problems and concerns in regards to bias and discrimination.
Does AI have bias?
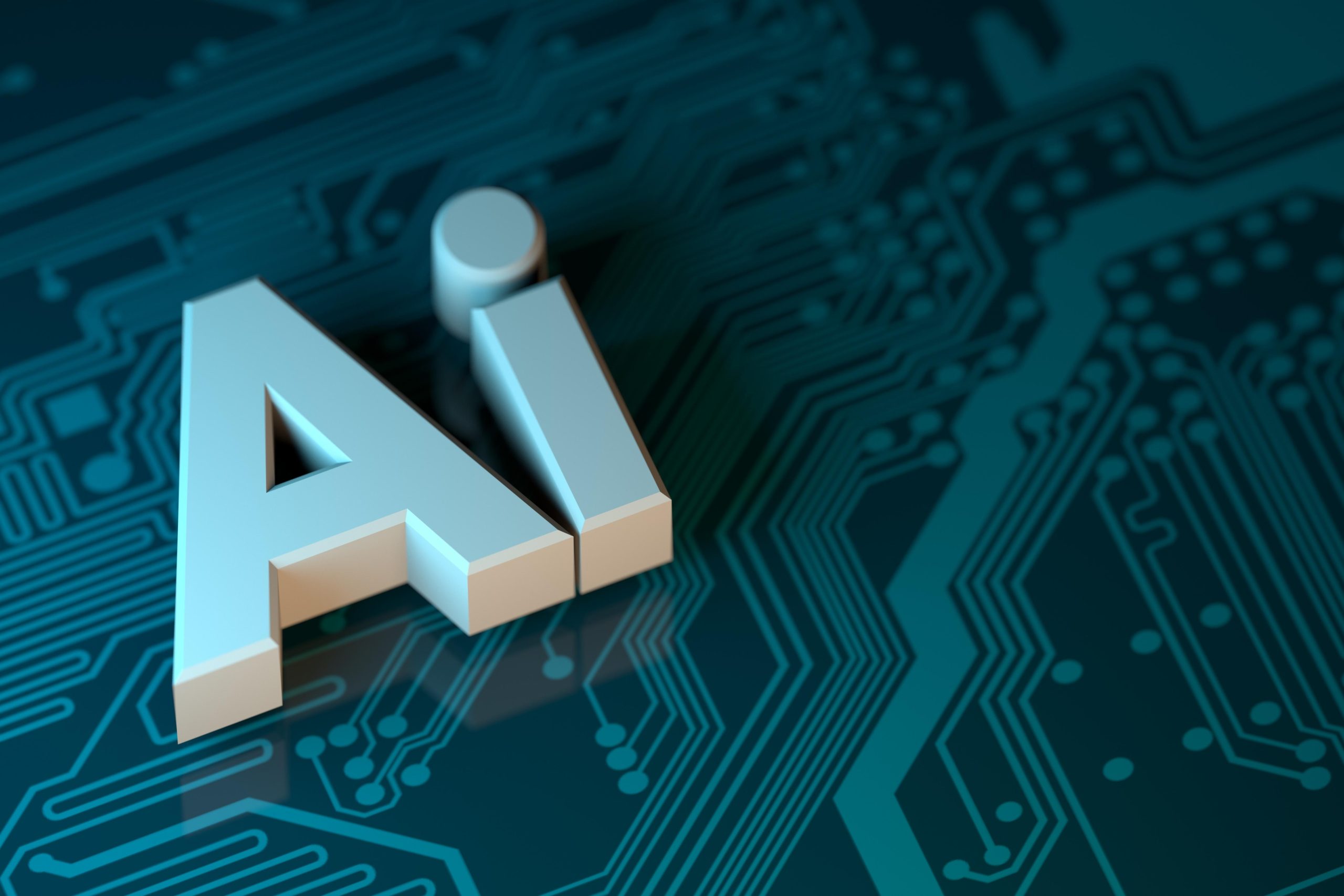
Because AI is programmed by humans and learns from human behaviors, bias and discrimination in AI does exist. AI depends on algorithms and data to do its “thinking” and to draw conclusions. If the algorithms or data are biased in any way, then the output will perpetuate, and sometimes even amplify, the bias.
What causes bias in AI?
AI uses groups of algorithms to learn. An algorithm is defined by Oxford Languages as “a process or set of rules to be followed in calculations or other problem-solving operations, especially by a computer.” While an algorithm is programmed to execute specific functions when encountering a specific trigger, AI uses algorithms a little differently. The groups of algorithms are instead designed to learn from data sets that are used to train the AI, which then attempts to emulate human thought and intuition. In other words, AI uses algorithms to analyze and find patterns in the data to predict what a human would do in a particular scenario. It can modify algorithms or create new algorithms based on what it has learned from the data, allowing it to acquire and apply knowledge and skills – the very definition of intelligence. AI bias occurs when AI gives results or makes decisions that reinforce stereotypes and either exclude or favor certain groups of people. If the data being used by algorithms is biased, then the algorithms will automate and perpetuate these biases.
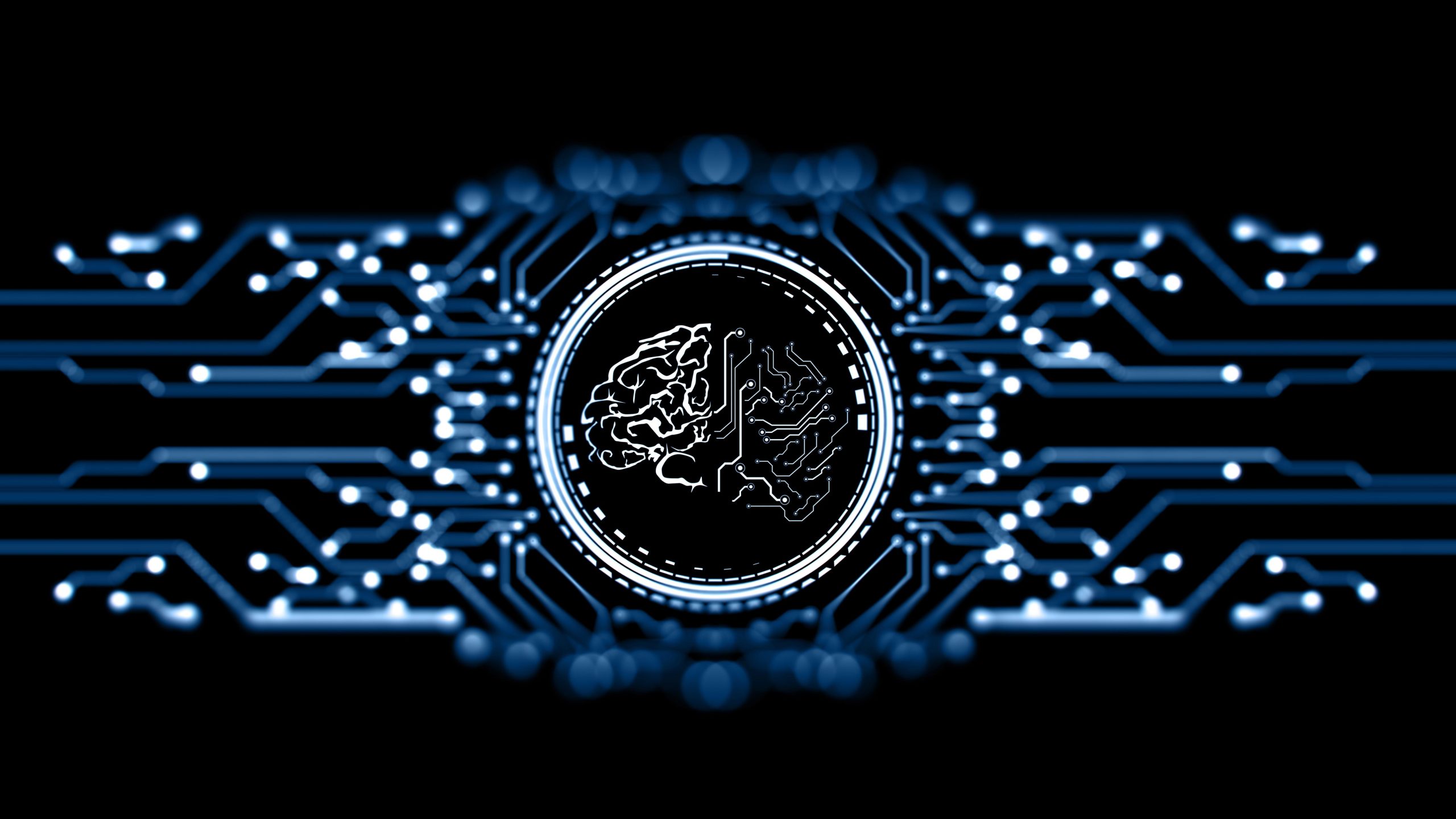
How does AI lead to discrimination?
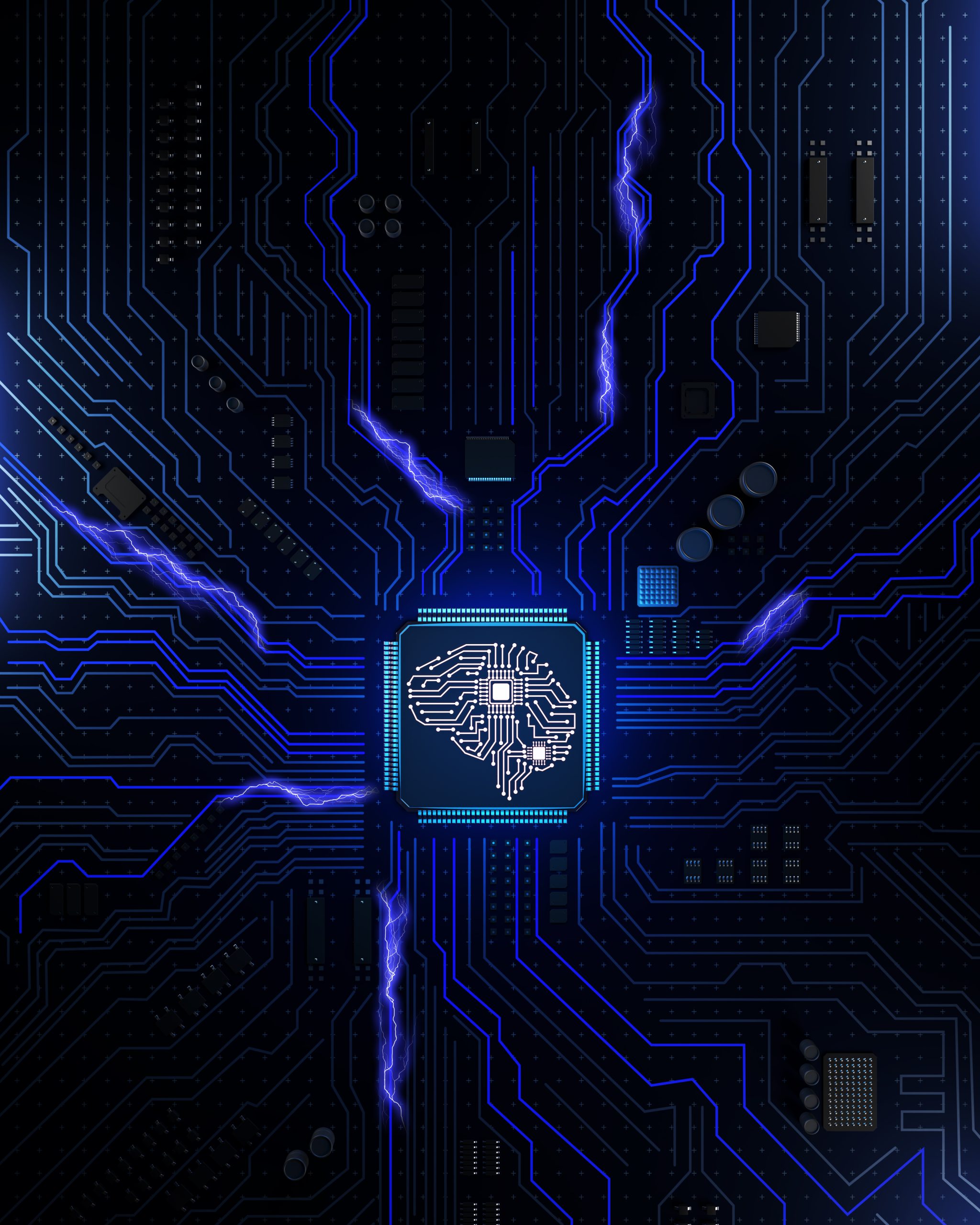
Although AI can change and adapt based on the data it’s given, it doesn’t have the ability to think beyond the data it’s been trained on. Therefore, if the data shows patterns of bias and AI interprets the data in a biased way, discrimination can occur. As more and more organizations turn to AI software and tools to streamline various processes, it becomes increasingly important for them to monitor the decisions being made to ensure that they are free from bias and discrimination.
The hiring process is one area in particular that businesses have progressively increased their AI use. There are many benefits of using AI in recruitment and selection, with the biggest advantage being the amount of time it can save recruiters and hiring managers. AI software can screen and source candidates by doing keyword searches to identify those that would potentially be the most qualified. AI is used in the interview process through AI assessments and tools that can act as preliminary or first interviews of sorts to narrow the pool down even more. There are even AI interview bots that conduct interviews and make hiring decisions.
While all of these tools can aid in making the recruitment and selection process quicker and more efficient, it can also lead to AI discrimination in hiring. AI software that is designed to scan resumes for specific keywords, skills, or qualifications may exclude people who are qualified but don’t fit the exact specifications. This could lead to applicants being discriminated against based on gender, age, race, or disabilities. Since these algorithms can also be programmed to eliminate applicants with gaps in their work experience, it can exclude competent candidates who have had to stop working due to a disability or illness or who took time off to be a stay-at-home caregiver. AI software that only scours the internet to source potential candidates can fail to identify quality contenders who don’t have much of an online presence. AI interviews typically record applicants and analyze everything from facial expressions, body language, and gestures to speech patterns and word choice. As a result, applicants with cultural speaking differences or a disability such as a speech impediment could be rated lower and screened out. Additionally, older adults or people with disabilities that impair cognitive or processing functions could have difficulty navigating the technology of an AI interview, resulting in them being removed from the pool of qualified candidates. All of these scenarios are a problem in and of themselves, but what is even more worrisome is that AI discrimination can become magnified if it then learns to eliminate future candidates because they have similar profiles to the ones that have already been screened out.
How do you mitigate bias in artificial intelligence?
According to a 2022 study done by DataRobot, AI bias is a growing concern for many of the polled technology leaders, which included Chief Information Officers, IT directors and managers, data scientists, and development leads from both the United States and the United Kingdom. The AI bias statistics gleaned from the surveys are telling, as over half (54%) of the respondents were very or extremely concerned about AI bias, and the majority (81%) would like more government regulation to define and prevent AI bias.
AI bias research has recently placed a lot of emphasis on bias mitigation strategies in an effort to address these concerns and reduce the amount of bias and discrimination that are occurring with AI. A number of technology experts and university researchers have proposed solutions to help minimize AI bias. One way is to review AI training data and test it using real-world applications. This will help ensure that the algorithms and data are representative of the types of people who it will actually be analyzing. Another way to mitigate bias is to have humans check (and recheck!) the decisions being made by AI. This helps ensure more transparency because a human can understand and explain how AI conclusions were drawn as well as being able to make changes to algorithms or data that could be perpetuating bias before it becomes a continuous loop. Proper AI training and education is another important piece that can make a difference in the way AI is utilized. Companies that use AI technology need to ensure that business and technology leaders, along with human resource departments, have the knowledge and understanding necessary to implement and interpret it, as well as how to recognize and prevent AI bias. It is also vital for companies to continuously be monitoring and auditing AI to confirm that the results and decisions are reasonable and unbiased. The Equal Employment Opportunity Commission (EEOC) is a federal agency that was established to administer and enforce civil rights laws against workplace discrimination, and how AI is used in the hiring process has become a hot topic for the EEOC. It too has issued multiple documents that offer guidance as part of its Artificial Intelligence and Algorithmic Fairness Initiative. AI use is only increasing, so it is imperative that organizations take the steps necessary to eliminate, or at least reduce, AI bias.
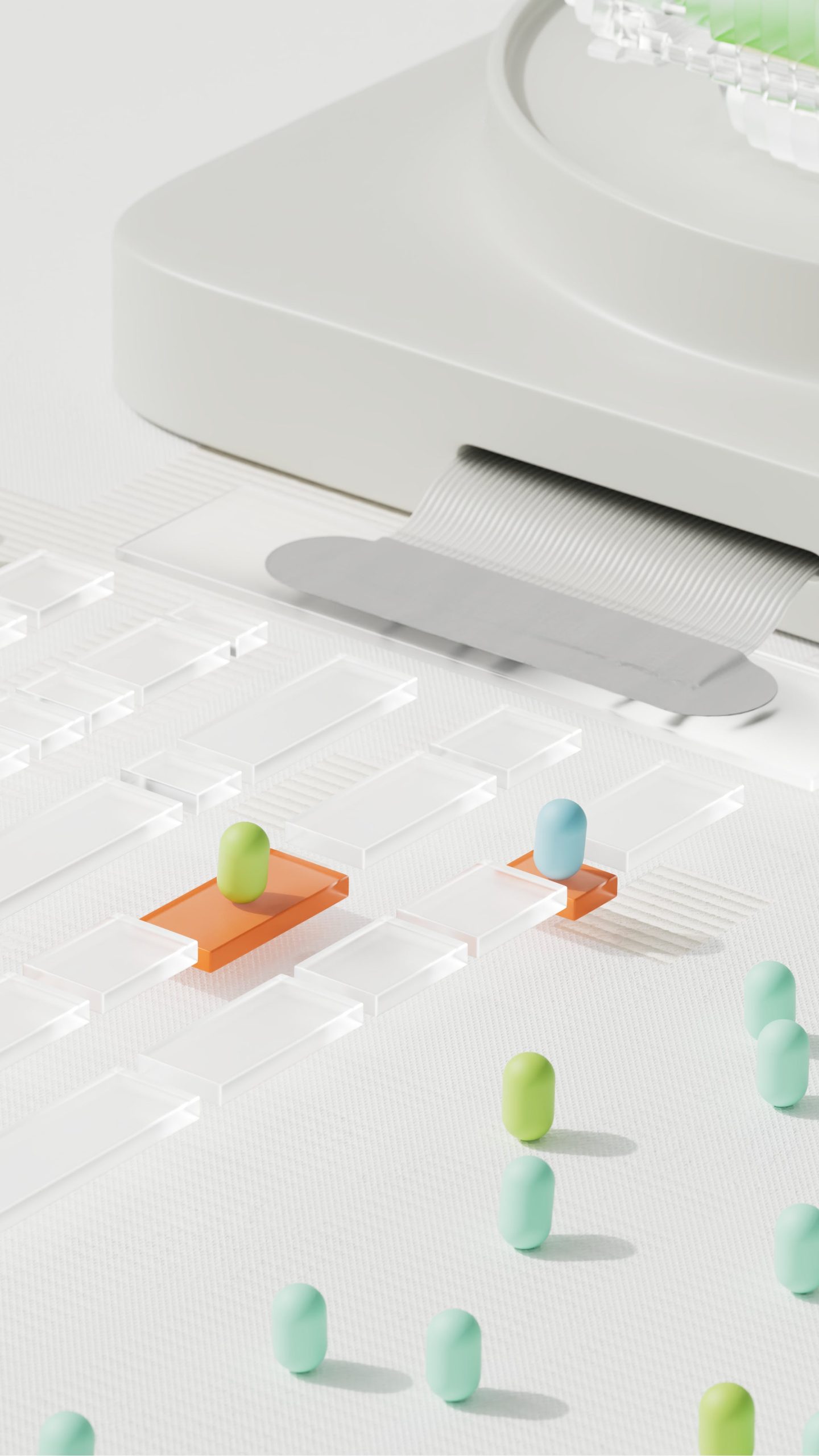
What are the three sources of bias in AI?
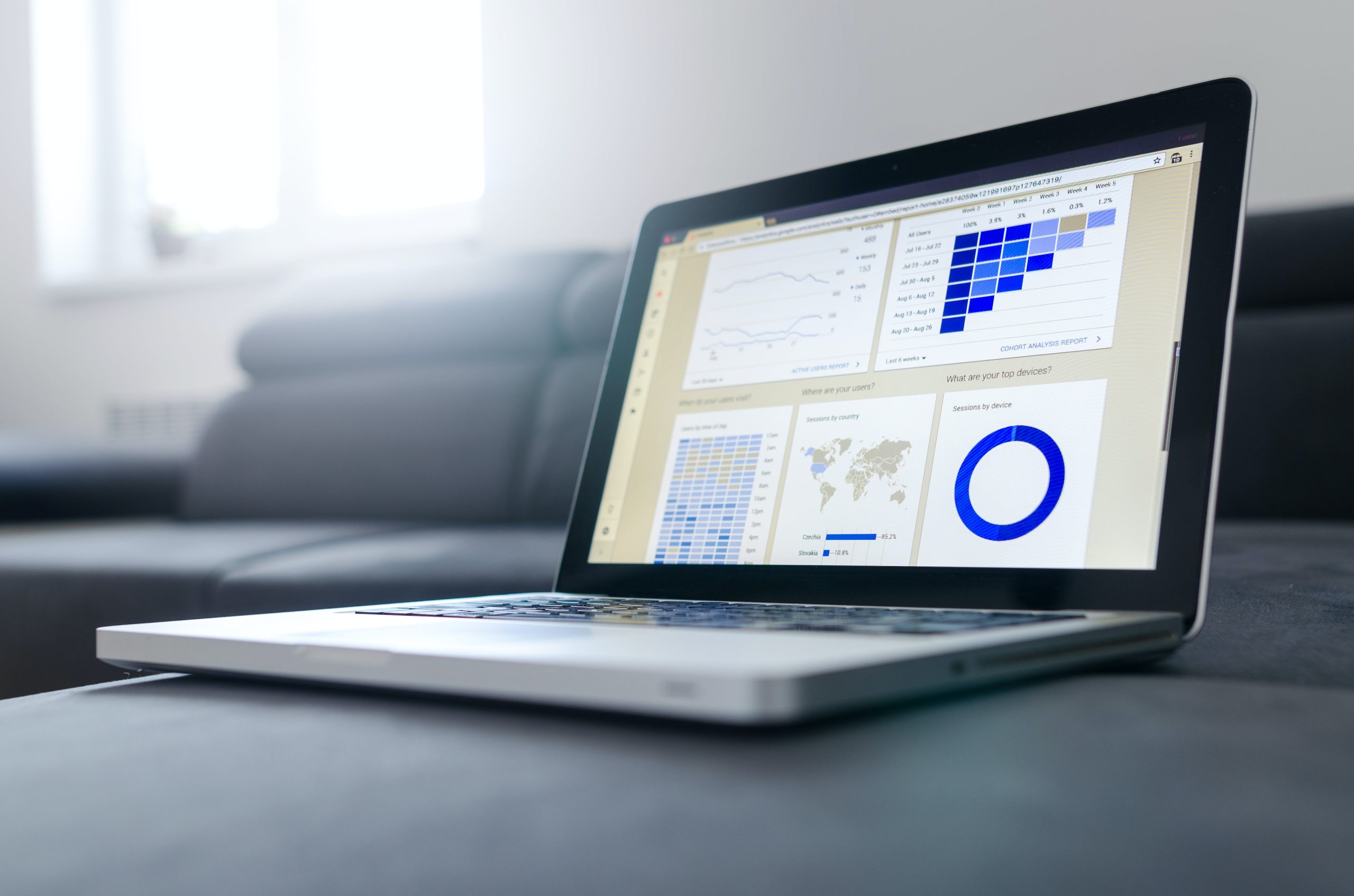
Researchers have identified three types of bias in AI: algorithmic, data, and human. Algorithmic bias refers to biases that occur within the design and implementation of an algorithm. It also includes biases that emerge when AI changes or adapts its algorithms based on data sets that result in inaccurate predictions or assumptions. An example of algorithmic bias would be Amazon’s recruiting algorithm that discriminated against women due to its analysis of past hiring practices. Data bias refers to bias that occurs when the data used to train AI is biased or is unrepresentative of a population as a whole. Researchers have found that some facial recognition software had difficulty recognizing people with darker-skin, in part because it was trained using data that was primarily made up of lighter-skinned individuals. Human bias, also called user bias, occurs when user generated data teaches AI, whether accidentally or intentionally, to be biased as a result of the way people interact with it. Microsoft’s chatbot, Tay, is an extreme example of this. It was designed to interact with the people of Twitter and learn through casual conversations. However, less than 24 hours after its launch, it was making and sharing discriminatory tweets based on interactions with users, many of whom were inundating Tay with messages of hate-mongering.
What are some recent examples of AI bias?
Some companies have had to learn the hard way about the negative effects of bias in AI. There are a few notable examples of AI bias within the last ten years. Probably one of the more notorious incidents occurred back in 2018 when news broke of Amazon’s secret recruiting system that discriminated against women. A team of engineers spent four years designing a recruiting program that would scan through resumes and identify the most qualified candidates. Unfortunately, the ten years of hiring data used to program the algorithm brought to light a pattern of gender inequality. As the majority of applicants had been men during this period, the algorithm considered females or resumes that used the words “women” or “women’s” less desirable and rated these resumes lower, thus leading to an AI hiring bias. The recruiting system was scrapped, and the team was disbanded then quietly erased that particular endeavor from their own resumes.
Court systems across the United States use a risk-assessment algorithm called Correctional Offender Management Profiling for Alternative Sanctions (COMPAS) as a tool when making sentencing decisions. COMPAS predicts the likelihood that the defendant will commit a crime again in the future. An investigation done by ProPublica claimed that an analysis of the predictions made by COMPAS found that it identified black defendants as having a higher reoffending rate and white defendants as being less likely to reoffend. They said, in fact, that black defendants were almost twice as likely as their white counterparts to be mislabeled as high-risk reoffenders but never go on to commit another crime. They also stated that the opposite was true for white defendants, who were often labeled lower-risk but had a higher rate of reoffending. Others have argued that this isn’t true, and COMPAS accurately predicts rates of reoffense for both black and white defendants at similar rates. COMPAS is still being used, but the debate continues about whether or not the predictions show racial bias.
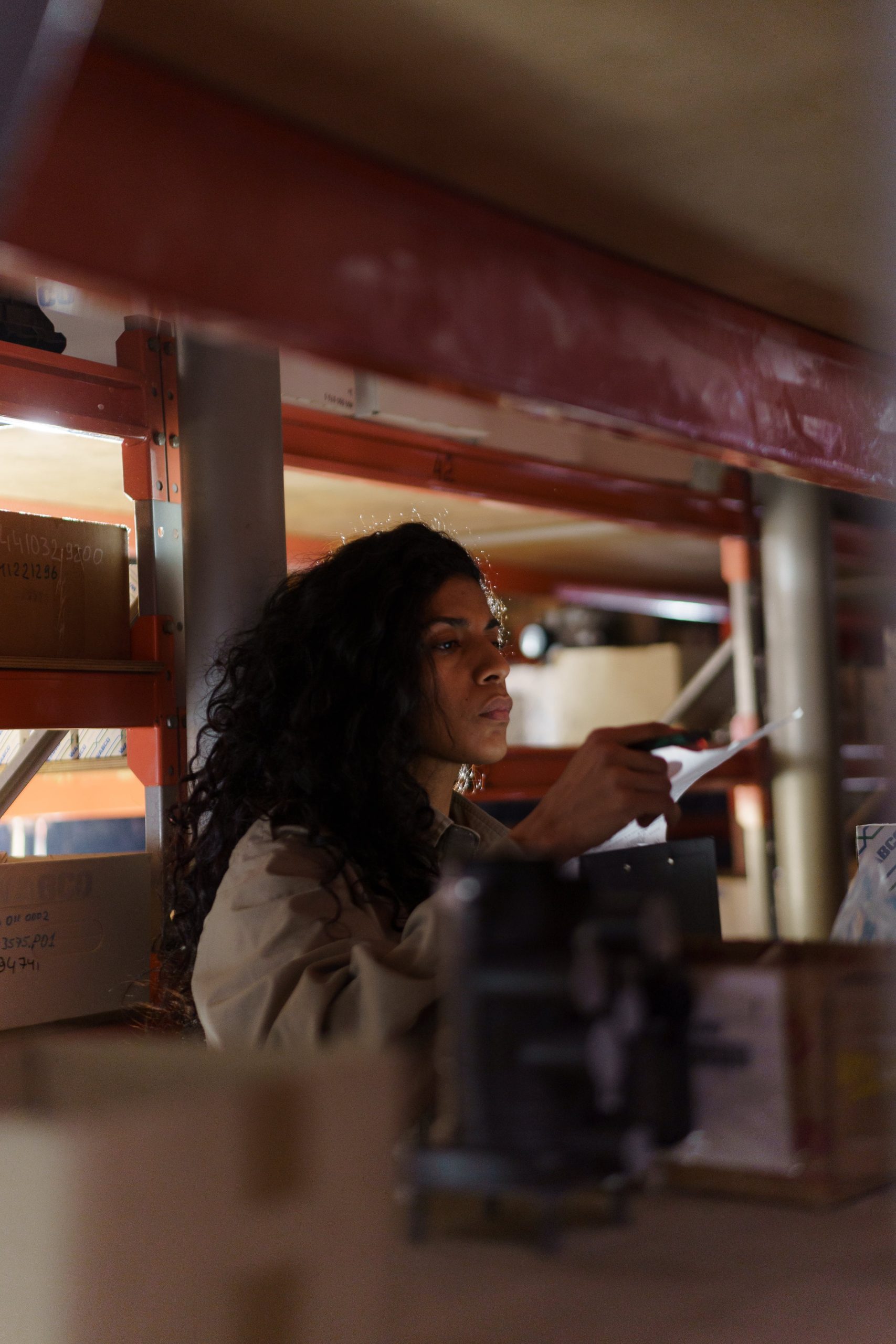
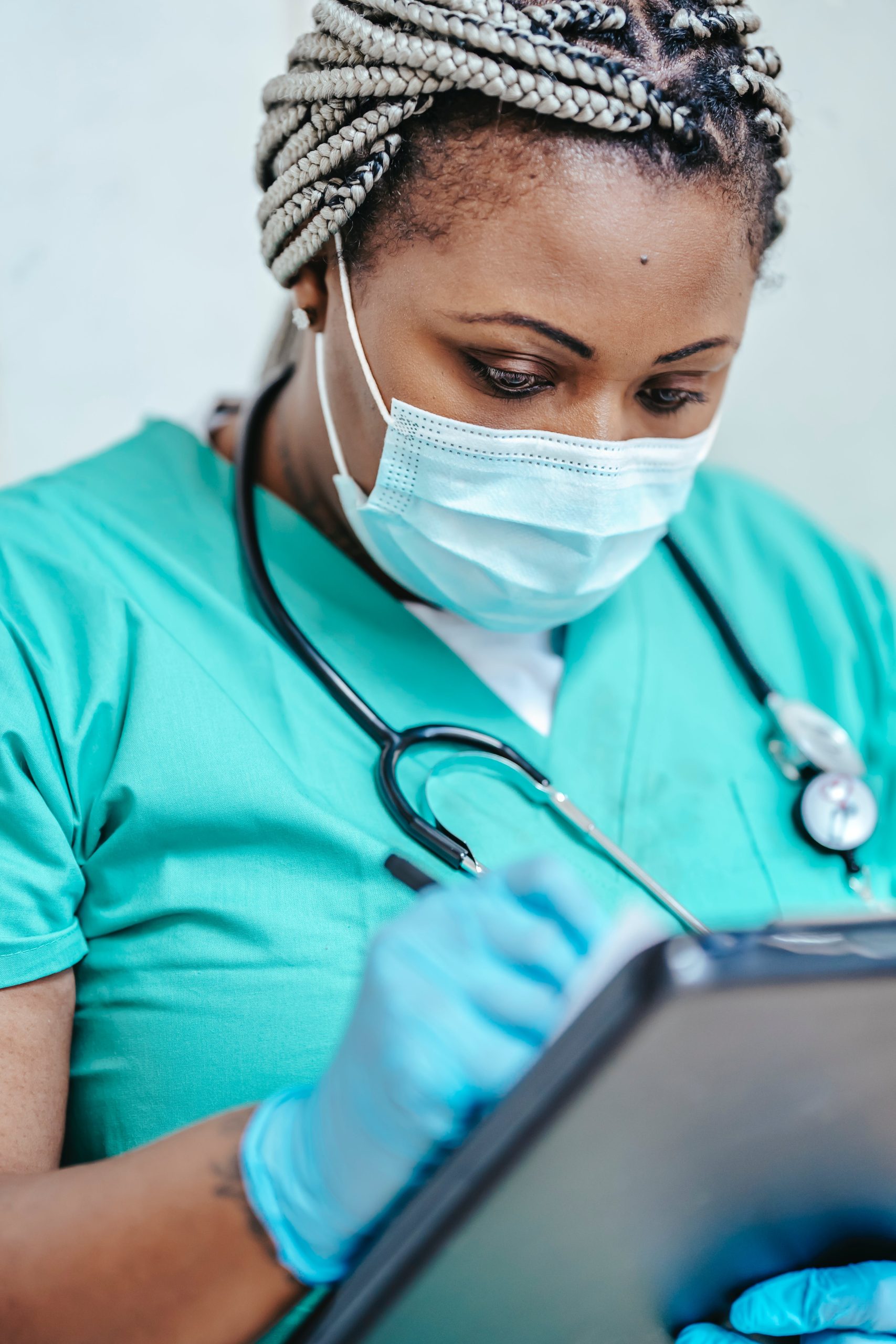
Another recent example of racial AI bias occurred in a healthcare algorithm designed to anticipate which patients might need extra care. This algorithm, used by many United States hospitals for over 200 million people, analyzed a patient’s previous healthcare costs to make these predictions, but because of the differences in the way that black and white patients pay for healthcare, it was miscalculating the risk scores. Researchers discovered that the algorithm was significantly favoring white patients over black patients and was assigning black people much lower risk scores than white people, putting sicker black patients on the same level as healthier white patients. As a result, black patients did not qualify for extra care as often as white ones, even if their needs were the same. Researchers worked with the company who had developed the healthcare algorithm to find variables other than cost history that could be used to assign risk factors, and they were able to reduce the bias by over 80 percent.
Social media giant, Facebook, has had its share of controversy, and in 2019 they found themselves facing a lawsuit for the way advertisers were permitted to target or exclude certain groups based on their race, gender, age, or religion. Women were singled out for employment advertisements pertaining to nursing or secretarial positions but were excluded from seeing other types of job ads that favored men as candidates. Older adults were also prevented from seeing many of the employment opportunities being advertised. Advertisements for housing were found to exclude certain minority groups. This lawsuit resulted in Facebook advertisers no longer being able to target ads pertaining to housing, employment, or credit offers
Why is bias a problem in machine learning?
Machine learning (ML) is the subset of AI that focuses on the use of data and algorithms to improve computer performance by giving machines the ability to “learn” without specifically being programmed. Bias in ML is a problem because it is often unintentional but the consequences of it can be significant. Per the DataRobot results, over one-third (36%) of the organizations reported that bias has had a negative impact on their business. Depending on the types of ML systems and how they are implemented, this included lost revenue (62%), lost customers (61%), lost employees (43%), incurring legal fees (35%), and loss of customer trust (6%). Organizations need to take steps to ensure that the data being used to train ML systems is comprehensive and that the algorithms are not perpetuating or amplifying AI bias.
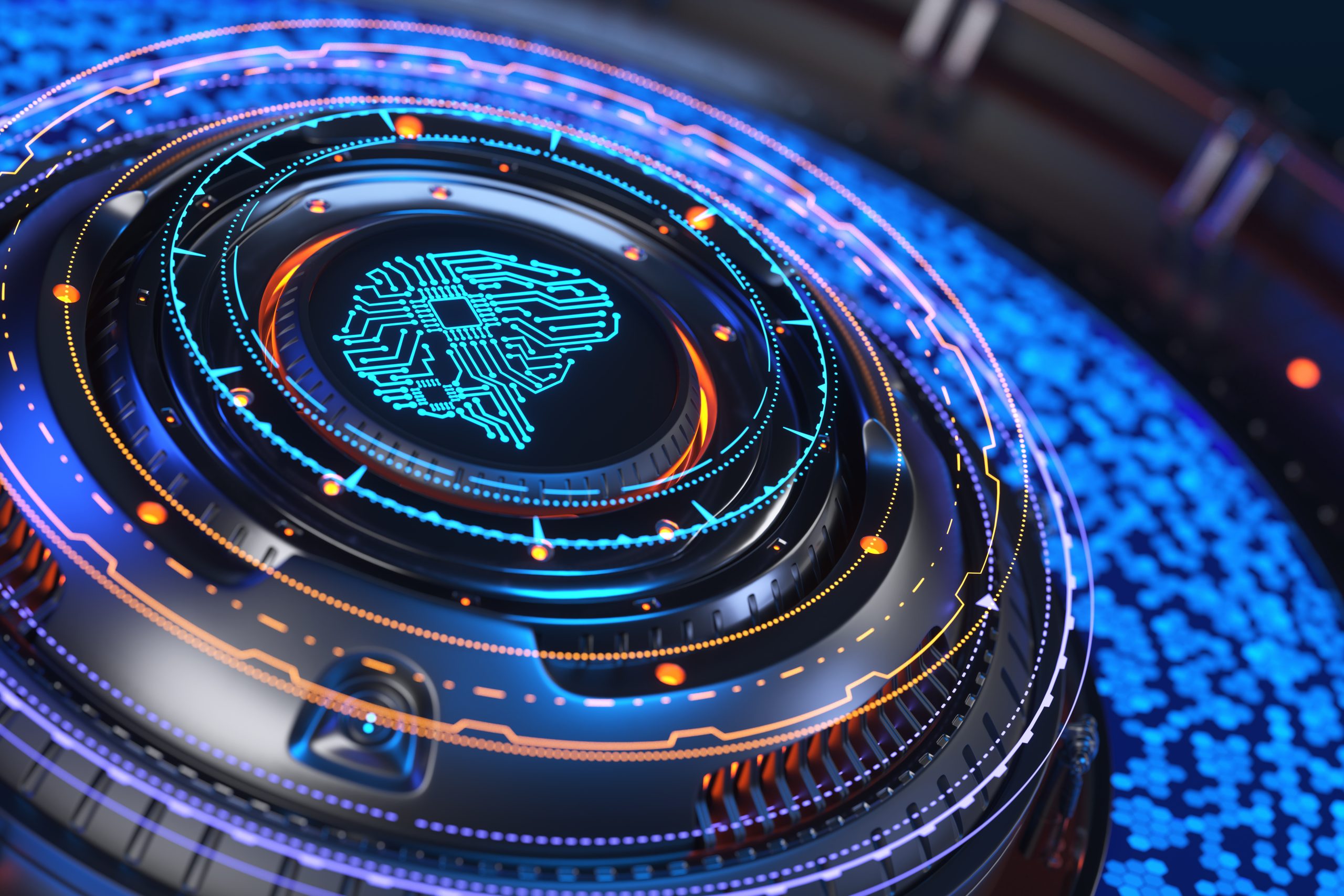